
Given the abundance of data in many real-world applications, graph nodes and edges could be annotated with multiple sets of attributes that could be derived from heterogeneous data sources. Graph clustering is one of the most important research topics in graph mining and network analysis. Other experiments show it is typically more than one order of magnitude faster than the state-of-the-art algorithms, which can only mine 0/1 matrices. Experiments on two real-world fuzzy tensors illustrate the versatility of the proposal. It builds upon multidupehack, a generalist pattern mining framework, which is now able to efficiently list skypatterns in addition to enforcing constraints on them. After explaining why and how their common mathematical property enables a safe pruning of the search space, an algorithm is presented.

The proposed solution supports a large class of measures. This article tackles the search of the skypatterns in a more general context than the 0/1 (aka Boolean) matrix: the fuzzy tensor. Skypatterns are Pareto-optimal patterns: no other pattern scores better on one of the chosen measures and scores at least as well on every remaining measure. Skypatterns were introduced to allow analysts to simply define the measures of interest, and to get as a result a set of globally optimal and semantically relevant patterns. Until recently, most algorithms have only handled constraints in an efficient way, i.e., every measure had to be associated with a user-defined threshold, which can be tricky to determine. To identify the patterns of interest in a dataset, an analyst may define several measures that score, in different ways, the relevance of a pattern. Many data mining tasks rely on pattern mining. The performance of the proposed technique is evaluated by using real-life as well The importance of a subgraph is determined by: (i) the order of the subgraph (the number of vertices)Īnd (ii) the subgraph edge connectivity. In this paper, we proposeĪ methodology to support such preferences by applying subgraph discovery in relational graphs towards retrieving importantĬonnected subgraphs. It would be moreĬonvenient for the user if “goodness” criteria could be set to evaluate the usefulness of these patterns, and if the userĬould provide preferences to the system regarding the characteristics of the discovered patterns.

May be large, and this number depends on the data characteristics and the frequency threshold specified.
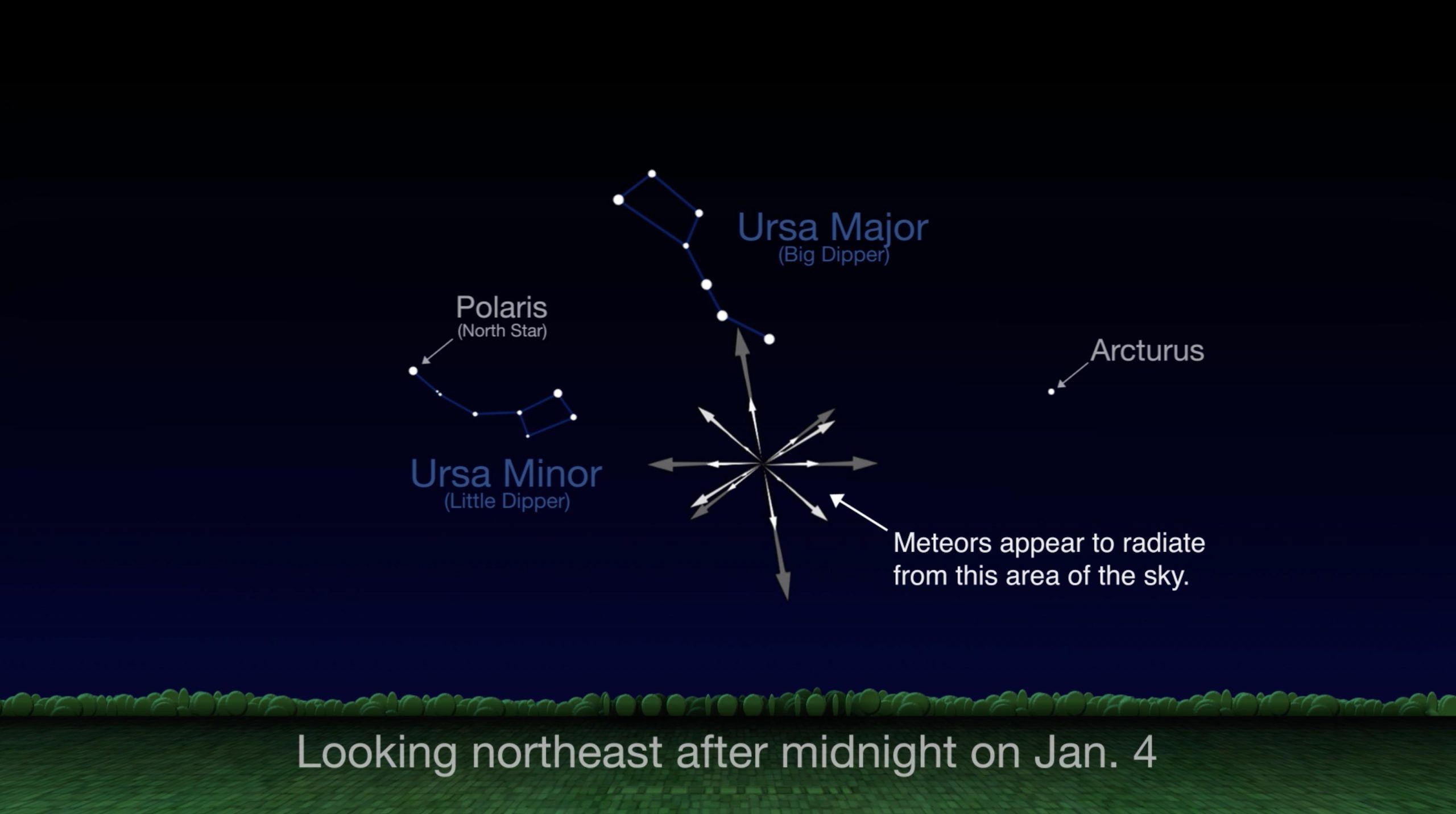
For example, applying frequent subgraph mining on a set of graphs the system returnsĪll connected subgraphs whose frequency is above a specified (usually user-defined) threshold. However, in some cases the number of mined patterns is large, posing difficulties Such as subgraphs that are likely to be useful. The basic approach followed by existing methods is to apply mining techniques on graph data to discover important patterns,

In a large graph representing a social network, (iii) analysis of transportation networks, (iv) community discovery in Webĭata. Some example applications are: (i) analysis of microarray data in bioinformatics, (ii) pattern discovery A significant number of applications require effective and efficient manipulation of relational graphs, towards discovering
